Tability is a cheatcode for goal-driven teams. Set perfect OKRs with AI, stay focused on the work that matters.
What are Machine Learning Team OKRs?
The Objective and Key Results (OKR) framework is a simple goal-setting methodology that was introduced at Intel by Andy Grove in the 70s. It became popular after John Doerr introduced it to Google in the 90s, and it's now used by teams of all sizes to set and track ambitious goals at scale.
How you write your OKRs can make a huge difference on the impact that your team will have at the end of the quarter. But, it's not always easy to write a quarterly plan that focuses on outcomes instead of projects.
That's why we have created a list of OKRs examples for Machine Learning Team to help. You can use any of the templates below as a starting point to write your own goals.
If you want to learn more about the framework, you can read our OKR guide online.
The best tools for writing perfect Machine Learning Team OKRs
Here are 2 tools that can help you draft your OKRs in no time.
Tability AI: to generate OKRs based on a prompt
Tability AI allows you to describe your goals in a prompt, and generate a fully editable OKR template in seconds.
- 1. Create a Tability account
- 2. Click on the Generate goals using AI
- 3. Describe your goals in a prompt
- 4. Get your fully editable OKR template
- 5. Publish to start tracking progress and get automated OKR dashboards
Watch the video below to see it in action 👇
Tability Feedback: to improve existing OKRs
You can use Tability's AI feedback to improve your OKRs if you already have existing goals.
- 1. Create your Tability account
- 2. Add your existing OKRs (you can import them from a spreadsheet)
- 3. Click on Generate analysis
- 4. Review the suggestions and decide to accept or dismiss them
- 5. Publish to start tracking progress and get automated OKR dashboards
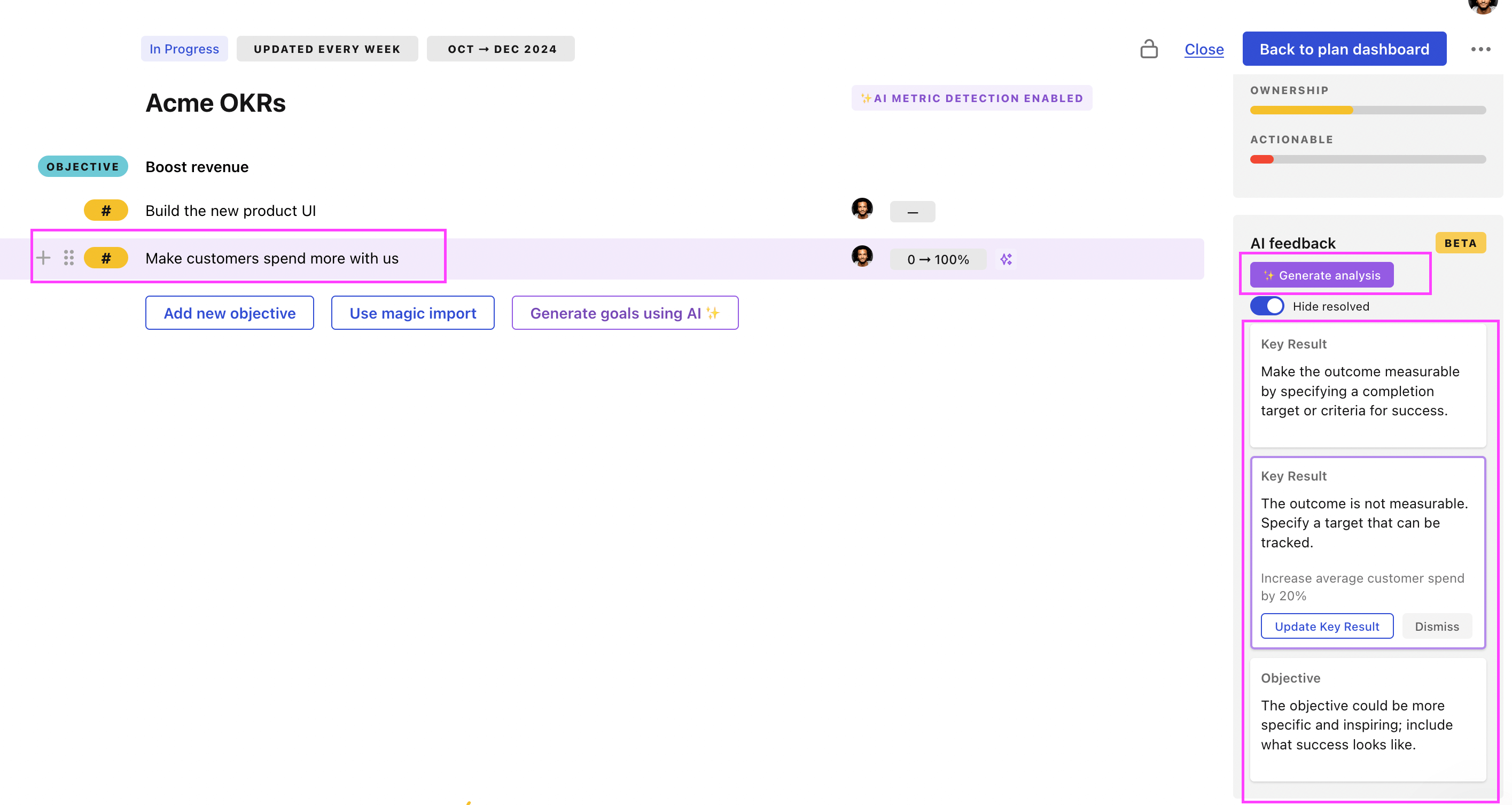
Tability will scan your OKRs and offer different suggestions to improve them. This can range from a small rewrite of a statement to make it clearer to a complete rewrite of the entire OKR.
Machine Learning Team OKRs examples
We've added many examples of Machine Learning Team Objectives and Key Results, but we did not stop there. Understanding the difference between OKRs and projects is important, so we also added examples of strategic initiatives that relate to the OKRs.
Hope you'll find this helpful!
OKRs to enhance machine learning model performance
ObjectiveEnhance machine learning model performance
KRAchieve 90% precision and recall in classifying test data
Implement and train various classifiers on the dataset
Evaluate and iterate model's performance using precision-recall metrics
Enhance the algorithm through machine learning tools and techniques
KRReduce model's prediction errors by 10%
Increase the versatility of training data
Evaluate and fine-tune model’s hyperparameters
Incorporate new relevant features into the model
KRIncrease model's prediction accuracy by 15%
Enhance data preprocessing and feature engineering methods
Implement advanced model optimization strategies
Validate model's performance using different datasets
OKRs to enhance global issue feedback classification accuracy and coverage
ObjectiveEnhance global issue feedback classification accuracy and coverage
KRReduce incorrect feedback classification cases by at least 25%
Train staff on best practices in feedback classification
Implement and continuously improve an automated classification system
Analyze and identify patterns in previous misclassifications
KRImprove machine learning model accuracy for feedback classification by 30%
Introduce a more complex, suitable algorithm or ensemble methods
Implement data augmentation to enhance the training dataset
Optimize hyperparameters using GridSearchCV or RandomizedSearchCV
KRExpand feedback coverage to include 20 new globally-relevant issues
Identify 20 globally-relevant issues requiring feedback
Develop a comprehensive feedback form for each issue
Roll out feedback tools across all platforms
OKRs to enhance platform steadiness via machine learning techniques
ObjectiveEnhance platform steadiness via machine learning techniques
KRReduce system downtime by 25% through predictive maintenance models
Implement predictive maintenance software with AI capabilities
Continuously monitor and optimize the predictive model performance
Train staff on utilizing the predictive maintenance model
KRIncrease system load capacity by 15% using optimization techniques
Optimize application/database code to improve performance
Upgrade hardware or increase server space
Identify system bottlenecks via comprehensive load testing
KRImplement ML algorithm to identify and resolve 30% more anomalies automatically
Train model to identify and categorize anomalies
Implement algorithm into existing systems for automated resolution
Develop a machine learning model for anomaly detection
OKRs to become an expert in large language models
ObjectiveBecome an expert in large language models
KRDemonstrate proficiency in implementing and fine-tuning large language models through practical projects
Continuously update and optimize large language models based on feedback and results obtained
Complete practical projects that showcase your proficiency in working with large language models
Create a large language model implementation plan and execute it efficiently
Identify areas of improvement in large language models and implement necessary fine-tuning
KRComplete online courses on large language models with a score of 90% or above
KREngage in weekly discussions or collaborations with experts in the field of large language models
Schedule a weekly video conference with language model experts
Document key insights and lessons learned from each discussion or collaboration
Share the findings and new knowledge with the team after each engagement
Prepare a list of discussion topics to cover during the collaborations
KRPublish two blog posts sharing insights and lessons learned about large language models
OKRs to launch machine learning product on website
ObjectiveLaunch machine learning product on website
KRGenerate at least 100 sign-ups for the machine learning product through website registration
Collaborate with influencers or industry experts to promote the machine learning product
Implement targeted online advertising campaigns to drive traffic to the website
Optimize website registration page to increase conversion rate
Run referral programs and offer incentives to encourage users to refer others
KRGenerate a revenue of $50,000 from sales of the machine learning product
Implement effective online advertising and social media campaigns to reach potential customers
Identify target market and create a comprehensive marketing strategy for machine learning product
Train sales team and provide them with necessary resources to effectively promote machine learning product
Conduct market research to determine competitive pricing and set optimal price point
KRIncrease website traffic by 20% through targeted marketing campaigns
Optimize website content with relevant keywords to improve organic search rankings
Conduct extensive keyword research to identify high-performing search terms
Develop and implement targeted advertising campaigns on social media platforms
Collaborate with industry influencers to gain exposure and drive traffic to the website
KRAchieve a customer satisfaction rating of 4 out of 5 through user feedback surveys
Analyze feedback survey data to identify areas for improvement and prioritize actions
Continuously monitor customer satisfaction ratings and adjust strategies as needed for improvement
Implement changes and improvements based on user feedback to enhance customer satisfaction
Develop and distribute user feedback surveys to gather customer satisfaction ratings
OKRs to establish a proficient AI team with skilled ML engineers and product manager
ObjectiveEstablish a proficient AI team with skilled ML engineers and product manager
KRRecruit an experienced AI product manager with a proven track record
Reach out to AI professionals on LinkedIn
Post the job ad on AI and tech-focused job boards
Draft a compelling job description for the AI product manager role
KRConduct an effective onboarding program to integrate new hires into the team
Arrange team building activities to promote camaraderie
Develop a comprehensive orientation package for new hires
Assign mentors to guide newcomers in their roles
KRInterview and hire 5 qualified Machine Learning engineers
Conduct interviews and evaluate candidates based on benchmarks
Promote job vacancies on recruitment platforms and LinkedIn
Develop detailed job descriptions for Machine Learning engineer positions
OKRs to implement machine learning strategies to cut customer attrition
ObjectiveImplement machine learning strategies to cut customer attrition
KRDecrease monthly churn rate by 15% through the application of predictive insights
Prioritize customer retention strategies with predictive modeling
Enhance user engagement based on predictive insights
Implement predictive analytics for customer behavior patterns
KRImplement machine learning solutions in 85% of our customer-facing interactions
Develop and test relevant ML models for these interactions
Identify customer interactions where machine learning can be applied
Integrate ML models into the existing customer interface
KRIncrease accurate churn prediction rates by 25% with a refined machine learning model
Gather and analyze data for evaluating churn rates
Intensify machine learning training on accurate prediction
Implement and test refined machine learning model
OKRs to develop an accurate and efficient face recognition system
ObjectiveDevelop an accurate and efficient face recognition system
KRAchieve a 95% recognition success rate in challenging lighting conditions
KRIncrease recognition speed by 20% through software and hardware optimizations
Upgrade hardware components to enhance system performance for faster recognition
Collaborate with software and hardware experts to identify and implement further optimization techniques
Conduct regular system maintenance and updates to ensure optimal functionality and speed
Optimize software algorithms to improve recognition speed by 20%
KRImprove face detection accuracy by 10% through algorithm optimization and training data augmentation
Train the updated algorithm using the augmented data to enhance face detection accuracy
Implement necessary adjustments to optimize the algorithm for improved accuracy
Conduct a thorough analysis of the existing face detection algorithm
Augment the training data by increasing diversity, quantity, and quality
KRReduce false positives and negatives by 15% through continuous model refinement and testing
Increase training dataset by collecting more diverse and relevant data samples
Apply advanced anomaly detection techniques to minimize false positives and negatives
Implement regular model performance evaluation and metrics tracking for refinement
Conduct frequent A/B testing to optimize model parameters and improve accuracy
OKRs to enhance fraud detection and prevention in the payment system
ObjectiveEnhance fraud detection and prevention in the payment system
KRReduce the number of fraudulent transactions by 25% through enhanced system security
Invest in fraud detection and prevention software
Conduct regular cybersecurity audits and fixes
Implement advanced encryption techniques for payment transactions
KRImplement machine learning algorithms to increase fraud detection accuracy by 40%
Train the algorithms with historical fraud data
Select appropriate machine learning algorithms for fraud detection
Test and tweak models' accuracy to achieve a 40% increase
KRTrain staff on new security protocols to reduce manual errors by 30%
Monitor and evaluate reduction in manual errors post-training
Schedule mandatory training sessions for all staff
Develop comprehensive training on new security protocols
OKRs to incorporate AI and ML to innovate our solution suite
ObjectiveIncorporate AI and ML to innovate our solution suite
KRAchieve 5 client testimonials acknowledging the improved solutions powered by AI/ML
Reach out to clients for feedback on AI/ML-powered solutions
Develop a simple feedback collection form
Analyze feedback and generate testimonials
KRTrain 80% of technical team in AI/ML concepts to ensure proficient implementation
Schedule regular training programs for technological staff
Identify AI/ML experts for in-house training sessions
Evaluate progress through knowledge assessments
KRDevelop 3 AI-enhanced features in existing products, improving functionality by 20%
Validate and measure functionality improvements post-AI enhancement
Identify three products that could benefit from AI integration
Customize AI algorithms to enhance the selected product features
Machine Learning Team OKR best practices
Generally speaking, your objectives should be ambitious yet achievable, and your key results should be measurable and time-bound (using the SMART framework can be helpful). It is also recommended to list strategic initiatives under your key results, as it'll help you avoid the common mistake of listing projects in your KRs.
Here are a couple of best practices extracted from our OKR implementation guide 👇
Tip #1: Limit the number of key results
The #1 role of OKRs is to help you and your team focus on what really matters. Business-as-usual activities will still be happening, but you do not need to track your entire roadmap in the OKRs.
We recommend having 3-4 objectives, and 3-4 key results per objective. A platform like Tability can run audits on your data to help you identify the plans that have too many goals.
Tip #2: Commit to weekly OKR check-ins
Don't fall into the set-and-forget trap. It is important to adopt a weekly check-in process to get the full value of your OKRs and make your strategy agile – otherwise this is nothing more than a reporting exercise.
Being able to see trends for your key results will also keep yourself honest.
Tip #3: No more than 2 yellow statuses in a row
Yes, this is another tip for goal-tracking instead of goal-setting (but you'll get plenty of OKR examples above). But, once you have your goals defined, it will be your ability to keep the right sense of urgency that will make the difference.
As a rule of thumb, it's best to avoid having more than 2 yellow/at risk statuses in a row.
Make a call on the 3rd update. You should be either back on track, or off track. This sounds harsh but it's the best way to signal risks early enough to fix things.
Save hours with automated Machine Learning Team OKR dashboards
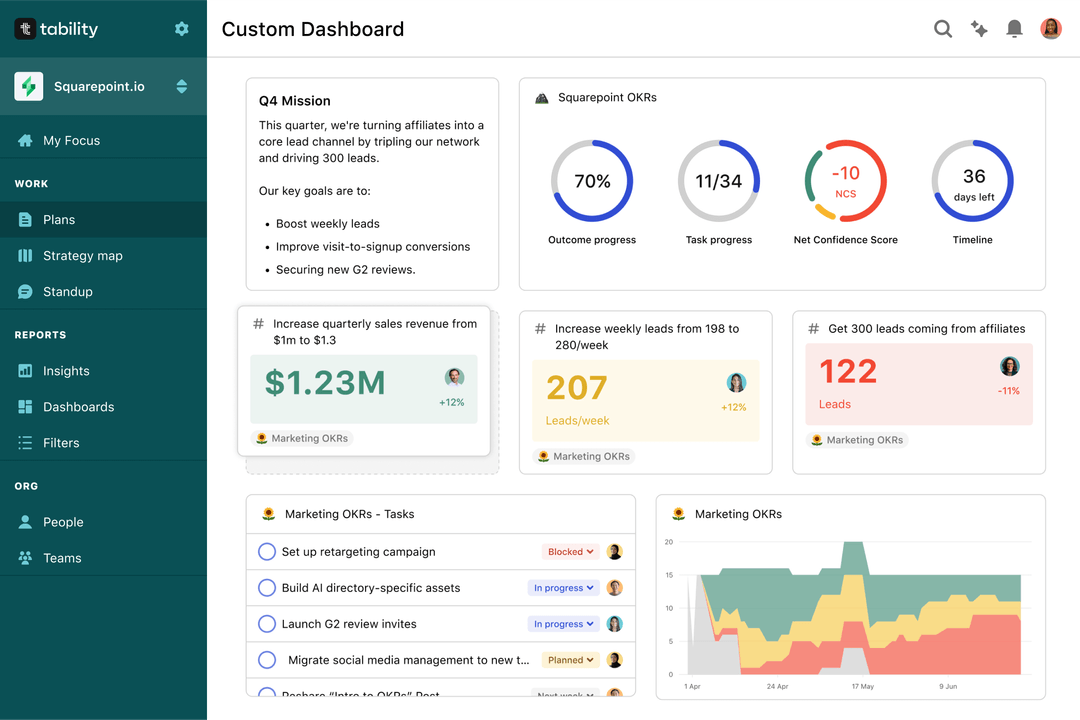
Quarterly OKRs should have weekly updates to get all the benefits from the framework. Reviewing progress periodically has several advantages:
- It brings the goals back to the top of the mind
- It will highlight poorly set OKRs
- It will surface execution risks
- It improves transparency and accountability
Spreadsheets are enough to get started. Then, once you need to scale you can use Tability to save time with automated OKR dashboards, data connectors, and actionable insights.
How to get Tability dashboards:
- 1. Create a Tability account
- 2. Use the importers to add your OKRs (works with any spreadsheet or doc)
- 3. Publish your OKR plan
That's it! Tability will instantly get access to 10+ dashboards to monitor progress, visualise trends, and identify risks early.
More Machine Learning Team OKR templates
We have more templates to help you draft your team goals and OKRs.
OKRs to turn users into true fans
OKRs to enhance collaboration and performance in the marketing team
OKRs to improve team responsiveness
OKRs to drive productivity and take the lead in software development initiatives
OKRs to instill a problem-solving mindset across the team
OKRs to enhance workflow efficiency and productivity