Tability is a cheatcode for goal-driven teams. Set perfect OKRs with AI, stay focused on the work that matters.
What are Data Science Team OKRs?
The OKR acronym stands for Objectives and Key Results. It's a goal-setting framework that was introduced at Intel by Andy Grove in the 70s, and it became popular after John Doerr introduced it to Google in the 90s. OKRs helps teams has a shared language to set ambitious goals and track progress towards them.
Formulating strong OKRs can be a complex endeavor, particularly for first-timers. Prioritizing outcomes over projects is crucial when developing your plans.
To aid you in setting your goals, we have compiled a collection of OKR examples customized for Data Science Team. Take a look at the templates below for inspiration and guidance.
If you want to learn more about the framework, you can read our OKR guide online.
The best tools for writing perfect Data Science Team OKRs
Here are 2 tools that can help you draft your OKRs in no time.
Tability AI: to generate OKRs based on a prompt
Tability AI allows you to describe your goals in a prompt, and generate a fully editable OKR template in seconds.
- 1. Create a Tability account
- 2. Click on the Generate goals using AI
- 3. Describe your goals in a prompt
- 4. Get your fully editable OKR template
- 5. Publish to start tracking progress and get automated OKR dashboards
Watch the video below to see it in action 👇
Tability Feedback: to improve existing OKRs
You can use Tability's AI feedback to improve your OKRs if you already have existing goals.
- 1. Create your Tability account
- 2. Add your existing OKRs (you can import them from a spreadsheet)
- 3. Click on Generate analysis
- 4. Review the suggestions and decide to accept or dismiss them
- 5. Publish to start tracking progress and get automated OKR dashboards
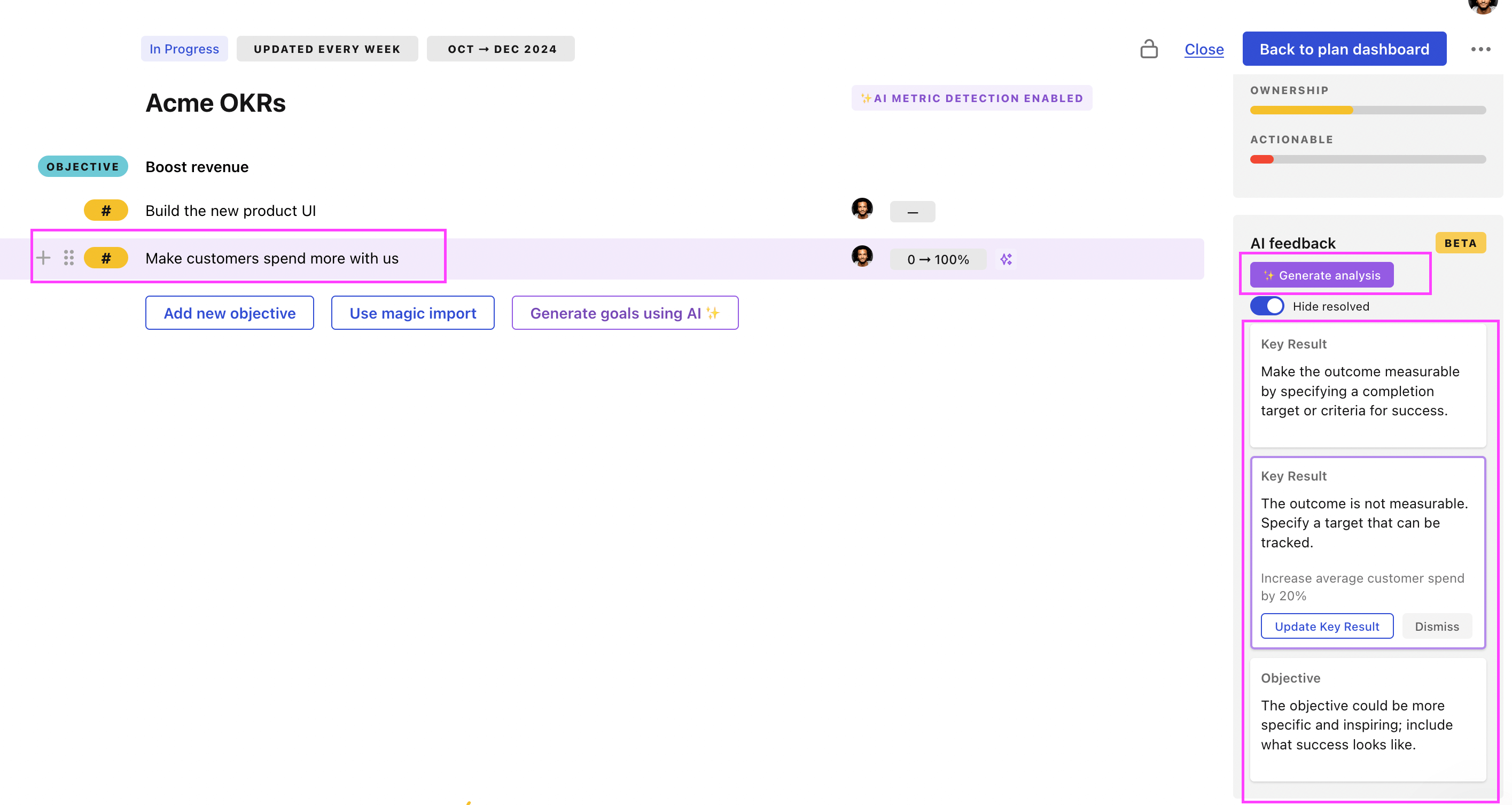
Tability will scan your OKRs and offer different suggestions to improve them. This can range from a small rewrite of a statement to make it clearer to a complete rewrite of the entire OKR.
Data Science Team OKRs examples
You will find in the next section many different Data Science Team Objectives and Key Results. We've included strategic initiatives in our templates to give you a better idea of the different between the key results (how we measure progress), and the initiatives (what we do to achieve the results).
Hope you'll find this helpful!
OKRs to implement MLOps system to enhance data science productivity and effectiveness
ObjectiveImplement MLOps system to enhance data science productivity and effectiveness
KRConduct training and enablement sessions to ensure team proficiency in utilizing MLOps tools
Organize knowledge-sharing sessions to enable cross-functional understanding of MLOps tool utilization
Provide hands-on practice sessions to enhance team's proficiency in MLOps tool
Create detailed documentation and resources for self-paced learning on MLOps tools
Schedule regular training sessions on MLOps tools for team members
KREstablish monitoring system to track model performance and detect anomalies effectively
Continuously enhance the monitoring system by incorporating feedback from stakeholders and adjusting metrics
Define key metrics and performance indicators to monitor and assess model performance
Establish a regular review schedule to analyze and address any detected performance anomalies promptly
Implement real-time monitoring tools and automate anomaly detection processes for efficient tracking
KRDevelop and integrate version control system to ensure traceability and reproducibility
Research available version control systems and their features
Identify the specific requirements and needs for the version control system implementation
Train and educate team members on how to effectively use the version control system
Develop a comprehensive plan for integrating the chosen version control system into existing workflows
KRAutomate deployment process to reduce time and effort required for model deployment
Research and select appropriate tools or platforms for automating the deployment process
Implement and integrate the automated deployment process into the existing model deployment workflow
Identify and prioritize key steps involved in the current deployment process
Develop and test deployment scripts or workflows using the selected automation tool or platform
OKRs to master fundamentals of Data Structures and Algorithms
ObjectiveMaster fundamentals of Data Structures and Algorithms
KRRead and summarize 3 books on advanced data structures and algorithms
Read each book thoroughly, highlighting important parts
Write summaries analyzing key concepts of each book
Purchase or borrow 3 books on advanced data structures and algorithms
KRComplete 10 online assignments on data structures with 90% accuracy
KRDevelop and successfully test 5 algorithms for complex mathematical problems
Implement and thoroughly test the devised algorithms
Develop unique algorithms to solve identified problems
Identify 5 complex mathematical problems requiring algorithms
OKRs to enhance effectiveness of future campaigns using predictive analytics
ObjectiveEnhance effectiveness of future campaigns using predictive analytics
KRSuccessfully implement predictive insights in 3 upcoming campaigns
Identify key goals and metrics for each campaign
Analyze insights and adjust campaign tactics accordingly
Integrate predictive analytics tools into campaign strategy
KRAchieve a 10% increase in campaign conversion rates through predictive analytics application
Analyze past campaigns data for forecasting
Deploy a predictive analytics tool in the campaign
Adjust marketing strategies based on predictions
KRIncrease predictive model accuracy to 85% by optimizing data sources and variables
Identify and integrate more relevant data sources
Perform feature selection to optimize variables
Regularly evaluate and refine the predictive model
OKRs to develop the skills and knowledge of junior data scientists
ObjectiveDevelop the skills and knowledge of junior data scientists
KREnhance junior data scientists' ability to effectively communicate insights through presentations and reports
Establish a feedback loop to continuously review and improve the communication skills of junior data scientists
Encourage junior data scientists to actively participate in team meetings and share their insights
Provide junior data scientists with training on effective presentation and report writing techniques
Assign a mentor to junior data scientists to guide and coach them in communication skills
KRIncrease junior data scientists' technical proficiency through targeted training programs
Provide hands-on workshops and projects to enhance practical skills of junior data scientists
Monitor and evaluate progress through regular assessments and feedback sessions
Develop customized training modules based on identified knowledge gaps
Conduct a skills assessment to identify knowledge gaps of junior data scientists
KRMeasure and improve junior data scientists' productivity by reducing their turnaround time for assigned tasks
KRFoster a supportive environment by establishing mentorship programs for junior data scientists
OKRs to enhance machine learning model performance
ObjectiveEnhance machine learning model performance
KRAchieve 90% precision and recall in classifying test data
Implement and train various classifiers on the dataset
Evaluate and iterate model's performance using precision-recall metrics
Enhance the algorithm through machine learning tools and techniques
KRReduce model's prediction errors by 10%
Increase the versatility of training data
Evaluate and fine-tune model’s hyperparameters
Incorporate new relevant features into the model
KRIncrease model's prediction accuracy by 15%
Enhance data preprocessing and feature engineering methods
Implement advanced model optimization strategies
Validate model's performance using different datasets
OKRs to enhance platform steadiness via machine learning techniques
ObjectiveEnhance platform steadiness via machine learning techniques
KRReduce system downtime by 25% through predictive maintenance models
Implement predictive maintenance software with AI capabilities
Continuously monitor and optimize the predictive model performance
Train staff on utilizing the predictive maintenance model
KRIncrease system load capacity by 15% using optimization techniques
Optimize application/database code to improve performance
Upgrade hardware or increase server space
Identify system bottlenecks via comprehensive load testing
KRImplement ML algorithm to identify and resolve 30% more anomalies automatically
Train model to identify and categorize anomalies
Implement algorithm into existing systems for automated resolution
Develop a machine learning model for anomaly detection
OKRs to enhance data-mining to generate consistent sales qualified leads
ObjectiveEnhance data-mining to generate consistent sales qualified leads
KRIncrease sales qualified leads generation by 30% through optimized data mining
Develop strategies to increase conversions by 30%
Optimize data collection to target potential customers
Implement advanced data mining techniques for lead generation
KRReduce false positives in lead generation by refining data mining process by 20%
Train staff in optimized data mining techniques
Evaluate current data mining practices for inefficiencies
Implement more accurate data filtering criteria
KRAchieve 90% accuracy in leads generated with improved data analysis algorithms
Regularly monitor and adjust algorithms to maintain accuracy
Develop enhanced data analysis algorithms for lead generation
Implement and test new algorithms on historical data
Data Science Team OKR best practices
Generally speaking, your objectives should be ambitious yet achievable, and your key results should be measurable and time-bound (using the SMART framework can be helpful). It is also recommended to list strategic initiatives under your key results, as it'll help you avoid the common mistake of listing projects in your KRs.
Here are a couple of best practices extracted from our OKR implementation guide 👇
Tip #1: Limit the number of key results
Having too many OKRs is the #1 mistake that teams make when adopting the framework. The problem with tracking too many competing goals is that it will be hard for your team to know what really matters.
We recommend having 3-4 objectives, and 3-4 key results per objective. A platform like Tability can run audits on your data to help you identify the plans that have too many goals.
Tip #2: Commit to weekly OKR check-ins
Setting good goals can be challenging, but without regular check-ins, your team will struggle to make progress. We recommend that you track your OKRs weekly to get the full benefits from the framework.
Being able to see trends for your key results will also keep yourself honest.
Tip #3: No more than 2 yellow statuses in a row
Yes, this is another tip for goal-tracking instead of goal-setting (but you'll get plenty of OKR examples above). But, once you have your goals defined, it will be your ability to keep the right sense of urgency that will make the difference.
As a rule of thumb, it's best to avoid having more than 2 yellow/at risk statuses in a row.
Make a call on the 3rd update. You should be either back on track, or off track. This sounds harsh but it's the best way to signal risks early enough to fix things.
Save hours with automated OKR dashboards
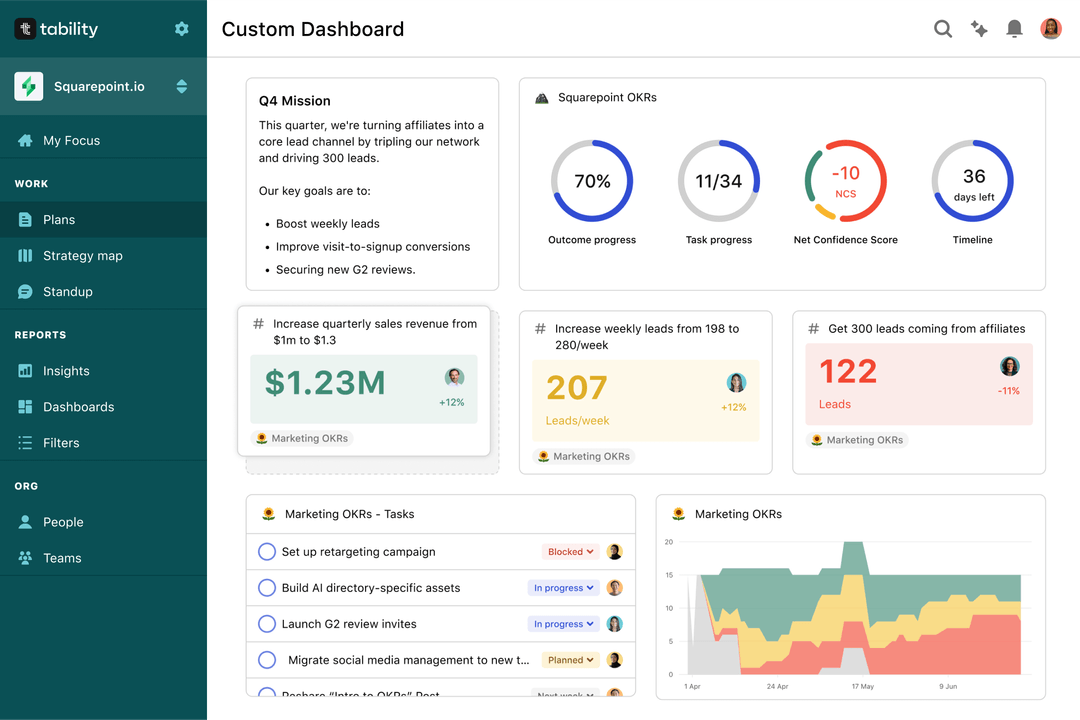
Your quarterly OKRs should be tracked weekly if you want to get all the benefits of the OKRs framework. Reviewing progress periodically has several advantages:
- It brings the goals back to the top of the mind
- It will highlight poorly set OKRs
- It will surface execution risks
- It improves transparency and accountability
Spreadsheets are enough to get started. Then, once you need to scale you can use Tability to save time with automated OKR dashboards, data connectors, and actionable insights.
How to get Tability dashboards:
- 1. Create a Tability account
- 2. Use the importers to add your OKRs (works with any spreadsheet or doc)
- 3. Publish your OKR plan
That's it! Tability will instantly get access to 10+ dashboards to monitor progress, visualise trends, and identify risks early.
More Data Science Team OKR templates
We have more templates to help you draft your team goals and OKRs.
OKRs to secure local channels and gain market insights
OKRs to increase savings towards the 10k annual goal
OKRs to broaden understanding of royalty components in contracts
OKRs to enhance overall customer satisfaction experience
OKRs to enhance web usability to boost conversions and lead quality
OKRs to increase overall audience engagement across all platforms