Use Tability to generate OKRs and initiatives in seconds.
tability.ioWhat are Data Quality Team OKRs?
The OKR acronym stands for Objectives and Key Results. It's a goal-setting framework that was introduced at Intel by Andy Grove in the 70s, and it became popular after John Doerr introduced it to Google in the 90s. OKRs helps teams has a shared language to set ambitious goals and track progress towards them.
Formulating strong OKRs can be a complex endeavor, particularly for first-timers. Prioritizing outcomes over projects is crucial when developing your plans.
To aid you in setting your goals, we have compiled a collection of OKR examples customized for Data Quality Team. Take a look at the templates below for inspiration and guidance.
If you want to learn more about the framework, you can read our OKR guide online.
Data Quality Team OKRs examples
You will find in the next section many different Data Quality Team Objectives and Key Results. We've included strategic initiatives in our templates to give you a better idea of the different between the key results (how we measure progress), and the initiatives (what we do to achieve the results).
Hope you'll find this helpful!
OKRs to enhance Data Quality
ObjectiveEnhance Data Quality
KRImprove data integrity by resolving critical data quality issues within 48 hours
KRIncrease accuracy of data by implementing comprehensive data validation checks
Train staff on proper data entry procedures to minimize errors and ensure accuracy
Regularly review and update data validation rules to match evolving requirements
Create a thorough checklist of required data fields and validate completeness
Design and implement automated data validation checks throughout the data collection process
KRAchieve a 90% completion rate for data cleansing initiatives across all databases
KRReduce data duplication by 20% through improved data entry guidelines and training
Establish a feedback system to receive suggestions and address concerns regarding data entry
Implement regular assessments to identify areas of improvement and address data duplication issues
Provide comprehensive training sessions on data entry guidelines for all relevant employees
Develop concise data entry guidelines highlighting key rules and best practices
OKRs to enhance the quality of data through augmented scrubbing techniques
ObjectiveEnhance the quality of data through augmented scrubbing techniques
KRTrain 80% of data team members on new robust data scrubbing techniques
Identify specific team members for training in data scrubbing
Schedule training sessions focusing on robust data scrubbing techniques
Conduct regular assessments to ensure successful training
KRReduce data scrubbing errors by 20%
Implement strict error-checking procedures in the data scrubbing process
Utilize automated data cleaning tools to minimize human errors
Provide comprehensive training on data scrubbing techniques to the team
KRImplement 3 new data scrubbing algorithms by the end of the quarter
Research best practices for data scrubbing algorithms
Design and code 3 new data scrubbing algorithms
Test and apply algorithms to existing data sets
OKRs to improve the overall quality of data across all departments
ObjectiveImprove the overall quality of data across all departments
KRReduce data inconsistencies by 20% through implementing a standardized data entry process
Implement uniform guidelines for data entry across all departments
Perform regular audits to maintain data consistency
Set up training sessions on standardized data entry procedures
KRIncrease data accuracy to 99% through rigorous data validation checks
Routinely monitor and correct data inconsistencies
Train staff on accurate data input methods
Implement a robust data validation system
KRDouble the number of regular data audits to ensure continued data quality
Identify current data audit frequency and benchmark
Communicate, implement, and track new audit plan
Establish new audit schedule with twice frequency
OKRs to enhance data quality and KPI report precision
ObjectiveEnhance data quality and KPI report precision
KRReduce data quality issues by 30% through regular quality checks and controls
Train team members on data quality control procedures
Develop a system for regular data quality checks
Implement corrective actions for identified data issues
KRImplement a streamlined process to avoid duplicated KPI reports by 50%
Create a standard template for all KPI reports
Implement a report review before distribution to check for duplications
Assign a single responsible person for finalizing reports
KRImprove report accuracy by 40% through stringent data verification protocols
Continually review and update protocols
Implement rigorous data verification protocols
Train staff on new verification procedures
OKRs to enhance data governance maturity with metadata and quality management
ObjectiveEnhance data governance maturity with metadata and quality management
KRImplement an enterprise-wide metadata management strategy in 75% of departments
Train department leads on the new metadata strategy implementation
Develop custom metadata strategy tailored to departmental needs
Identify key departments requiring metadata management strategy
KRDecrease data-related issues by 30% through improved data quality measures
Incorporate advanced data quality check software
Implement a rigorous data validation process
Offer periodic training on data management best practices
KRTrain 80% of the team on data governance and quality management concepts
Identify team members requiring data governance training
Conduct quality management training sessions
Schedule training on data governance concepts
OKRs to enhance metrics quality and interpretability
ObjectiveEnhance metrics quality and interpretability
KRImplement a metrics dashboard with simple, visually clear displays
Identify key metrics to track and display
Design a user-friendly dashboard layout
Code and test the dashboard for functionality
KRDevelop 5 additional relevant, actionable metrics by end of Q2
Implement and test performance metrics
Investigate potential key performance indicators
Design data collection methods for new metrics
KRIncrease the precision of metrics measurement by 15%
Review and improve current metrics measurement processes
Implement advanced analytics software for accurate data collection
Train staff on precise metrics measurement skills and techniques
OKRs to implement robust tracking of core Quality Assurance (QA) metrics
ObjectiveImplement robust tracking of core Quality Assurance (QA) metrics
KRDevelop an automated QA metrics tracking system within two weeks
Identify necessary metrics for quality assurance tracking
Research and select software for automation process
Configure software to track and report desired metrics
KRDeliver biweekly reports showing improvements in tracked QA metrics
Compile and submit a biweekly improvement report
Highlight significant improvements in collected QA data
Gather and analyze QA metrics data every two weeks
KRAchieve 100% accuracy in data capture on QA metrics by month three
OKRs to enhance Salesforce Lead Quality
ObjectiveEnhance Salesforce Lead Quality
KRImprove lead scoring accuracy by 10% through data enrichment activities
Analyze current lead scoring model efficiency
Implement strategic data enrichment techniques
Train team on data quality management
KRLower lead drop-off by 15% through better segmentation
Create personalized content for segmented leads
Implement a data-driven lead scoring system
Develop comprehensive profiles for ideal target customers
KRAchieve 20% increase in conversion rate of generated leads
Enhance lead qualification process to improve lead quality
Implement targeted follow-up strategies to reengage cold leads
Optimize landing page design to enhance user experience
OKRs to execute seamless Data Migration aligned with project plan
ObjectiveExecute seamless Data Migration aligned with project plan
KRTrain 85% of the team on new systems and data use by end of period
Monitor and document each member's training progress
Identify team members not yet trained on new systems
Schedule training sessions for identified team members
KRIdentify and document all data sources to migrate by end of Week 2
Create a list of all existing data sources
Document details of selected data sources
Assess and determine sources for migration
KRTest and validate data integrity post-migration with 100% accuracy
Develop a detailed data testing and validation plan
Execute data integrity checks after migration
Fix all detected data inconsistencies
OKRs to enhance data engineering capabilities to drive software innovation
ObjectiveEnhance data engineering capabilities to drive software innovation
KRImprove data quality by implementing automated data validation and monitoring processes
Implement chosen data validation tool
Research various automated data validation tools
Regularly monitor and assess data quality
KREnhance software scalability by optimizing data storage and retrieval mechanisms for large datasets
Optimize SQL queries for faster data retrieval
Adopt a scalable distributed storage system
Implement a more efficient database indexing system
KRIncrease data processing efficiency by optimizing data ingestion pipelines and reducing processing time
Develop optimization strategies for lagging pipelines
Implement solutions to reduce data processing time
Analyze current data ingestion pipelines for efficiency gaps
How to write your own Data Quality Team OKRs
1. Get tailored OKRs with an AI
You'll find some examples below, but it's likely that you have very specific needs that won't be covered.
You can use Tability's AI generator to create tailored OKRs based on your specific context. Tability can turn your objective description into a fully editable OKR template -- including tips to help you refine your goals.
- 1. Go to Tability's plan editor
- 2. Click on the "Generate goals using AI" button
- 3. Use natural language to describe your goals
Tability will then use your prompt to generate a fully editable OKR template.
Watch the video below to see it in action 👇
Option 2. Optimise existing OKRs with Tability Feedback tool
If you already have existing goals, and you want to improve them. You can use Tability's AI feedback to help you.
- 1. Go to Tability's plan editor
- 2. Add your existing OKRs (you can import them from a spreadsheet)
- 3. Click on "Generate analysis"
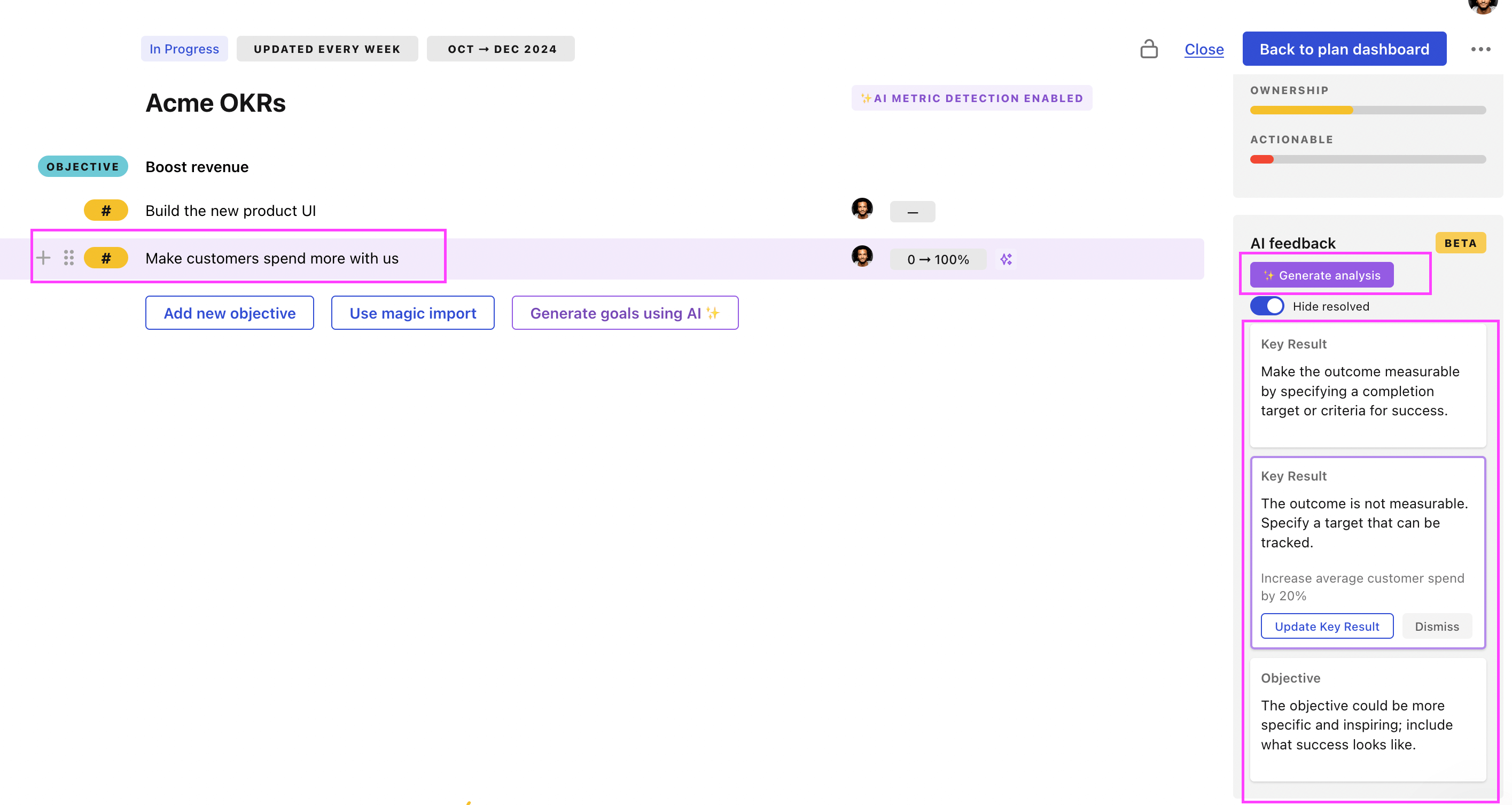
Tability will scan your OKRs and offer different suggestions to improve them. This can range from a small rewrite of a statement to make it clearer to a complete rewrite of the entire OKR.
You can then decide to accept the suggestions or dismiss them if you don't agree.
Option 3. Use the free OKR generator
If you're just looking for some quick inspiration, you can also use our free OKR generator to get a template.
Unlike with Tability, you won't be able to iterate on the templates, but this is still a great way to get started.
Data Quality Team OKR best practices
Generally speaking, your objectives should be ambitious yet achievable, and your key results should be measurable and time-bound (using the SMART framework can be helpful). It is also recommended to list strategic initiatives under your key results, as it'll help you avoid the common mistake of listing projects in your KRs.
Here are a couple of best practices extracted from our OKR implementation guide 👇
Tip #1: Limit the number of key results
Having too many OKRs is the #1 mistake that teams make when adopting the framework. The problem with tracking too many competing goals is that it will be hard for your team to know what really matters.
We recommend having 3-4 objectives, and 3-4 key results per objective. A platform like Tability can run audits on your data to help you identify the plans that have too many goals.
Tip #2: Commit to weekly OKR check-ins
Setting good goals can be challenging, but without regular check-ins, your team will struggle to make progress. We recommend that you track your OKRs weekly to get the full benefits from the framework.
Being able to see trends for your key results will also keep yourself honest.
Tip #3: No more than 2 yellow statuses in a row
Yes, this is another tip for goal-tracking instead of goal-setting (but you'll get plenty of OKR examples above). But, once you have your goals defined, it will be your ability to keep the right sense of urgency that will make the difference.
As a rule of thumb, it's best to avoid having more than 2 yellow/at risk statuses in a row.
Make a call on the 3rd update. You should be either back on track, or off track. This sounds harsh but it's the best way to signal risks early enough to fix things.
How to track your Data Quality Team OKRs
Your quarterly OKRs should be tracked weekly in order to get all the benefits of the OKRs framework. Reviewing progress periodically has several advantages:
- It brings the goals back to the top of the mind
- It will highlight poorly set OKRs
- It will surface execution risks
- It improves transparency and accountability
Spreadsheets are enough to get started. Then, once you need to scale you can use a proper OKR platform to make things easier.
If you're not yet set on a tool, you can check out the 5 best OKR tracking templates guide to find the best way to monitor progress during the quarter.
More Data Quality Team OKR templates
We have more templates to help you draft your team goals and OKRs.
OKRs to attain IIBA ECBA certification
OKRs to gain comprehensive understanding of Behavior Driven Development (BDD)
OKRs to enhance strategic thinking and adapt to changes effectively
OKRs to make business travel process fully digital
OKRs to boost talent retention
OKRs to elevate productivity in academic research